
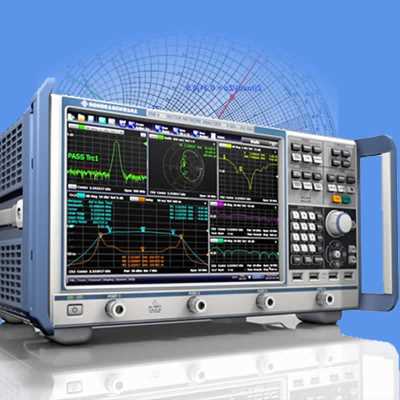
Making use of the bosonic quasiparticles 3, it offers low-power consuming wave-based computing with microwave signals up to THz frequencies 4, 5, 6. Magnonics which is based on charge-free angular momentum flow via spin waves (magnons), promises to overcome this dilemma. However, even for optical computers the currently implemented von-Neumann device architecture still suffers from the signal-conversion bottleneck, particularly in case of memory-intensive machine learning applications. Waves for data processing like photons in optical computers already avoid charge flow and excessive Joule heating at high clock frequencies. So far, such a storage device exploits reprogrammable states in a materials class which differs from the semiconductors and therefore requires signal conversion. The significant development slows down however due to overheating of processors at increased clock frequencies as well as the data-transfer bottleneck between charge-based processors and a nonvolatile storage device like a magnetic hard disk 1, 2. Combined with the already existing wave logic, our discovery is path-breaking for the new era of magnonics-based in-memory computation and beyond von Neumann computer architectures.Ĭharge-based electronics exploiting semiconductors and metals have led to the digital age and given rise to ever increasing data-processing power during the last decades. We show that the spin waves can reverse large arrays of ferromagnetic stripes at a strikingly small power level.
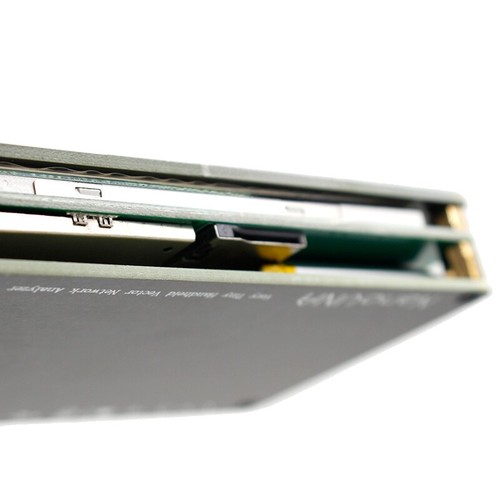
Thereby, the charge-free angular momentum flow is stored after transmission over a macroscopic distance. Here, we report the reversal of ferromagnetic nanostripes by spin waves which propagate in an underlying spin-wave bus. The conversion problem would be solved if spin wave amplitudes could be stored directly in a magnetic memory. Their angular momentum enables power-efficient computation without charge flow. There is a challenging quest for novel approaches to overcome this so-called von Neumann bottleneck.
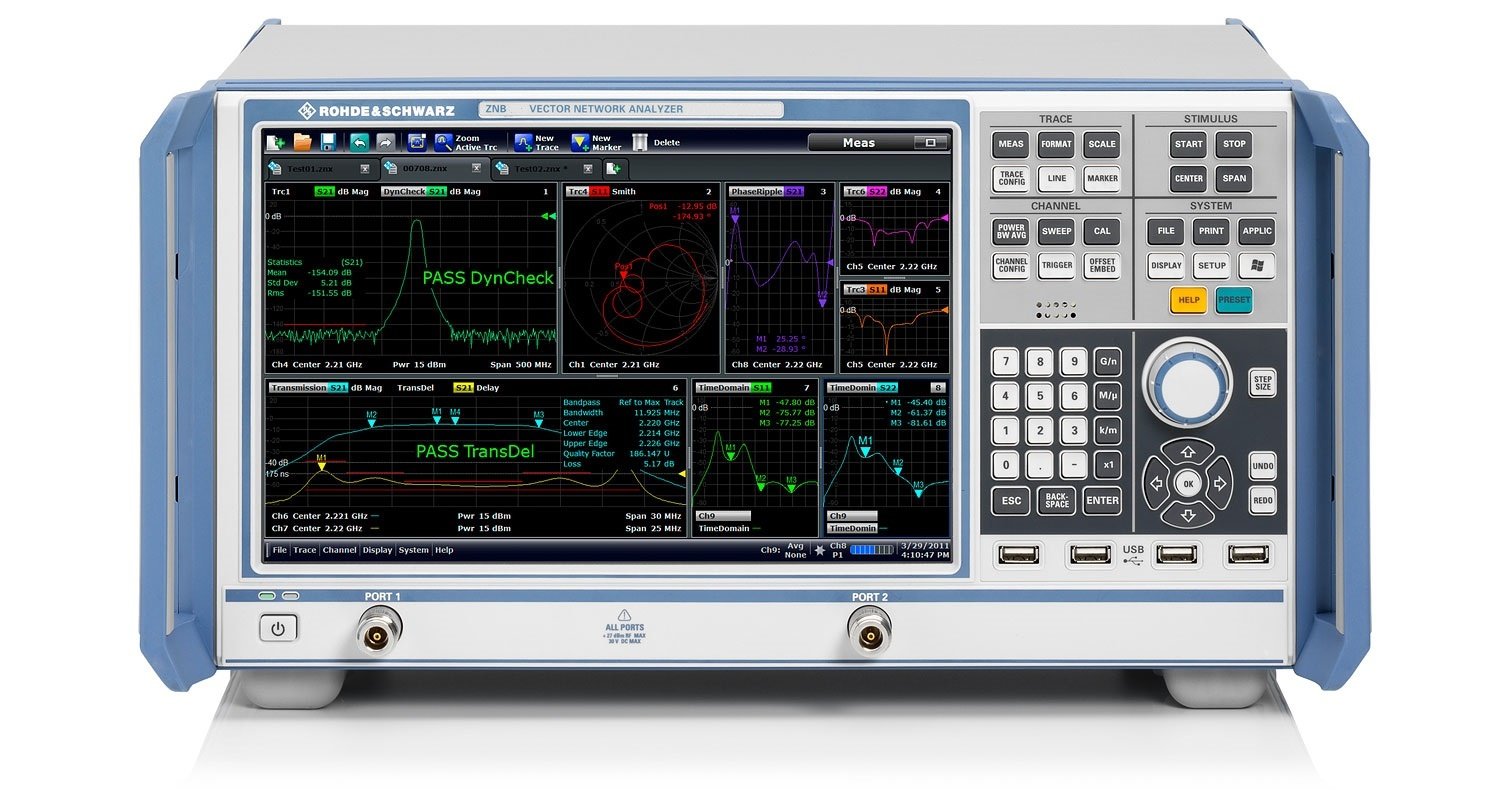
Despite the unprecedented downscaling of CMOS integrated circuits, memory-intensive machine learning and artificial intelligence applications are limited by data conversion between memory and processor.
